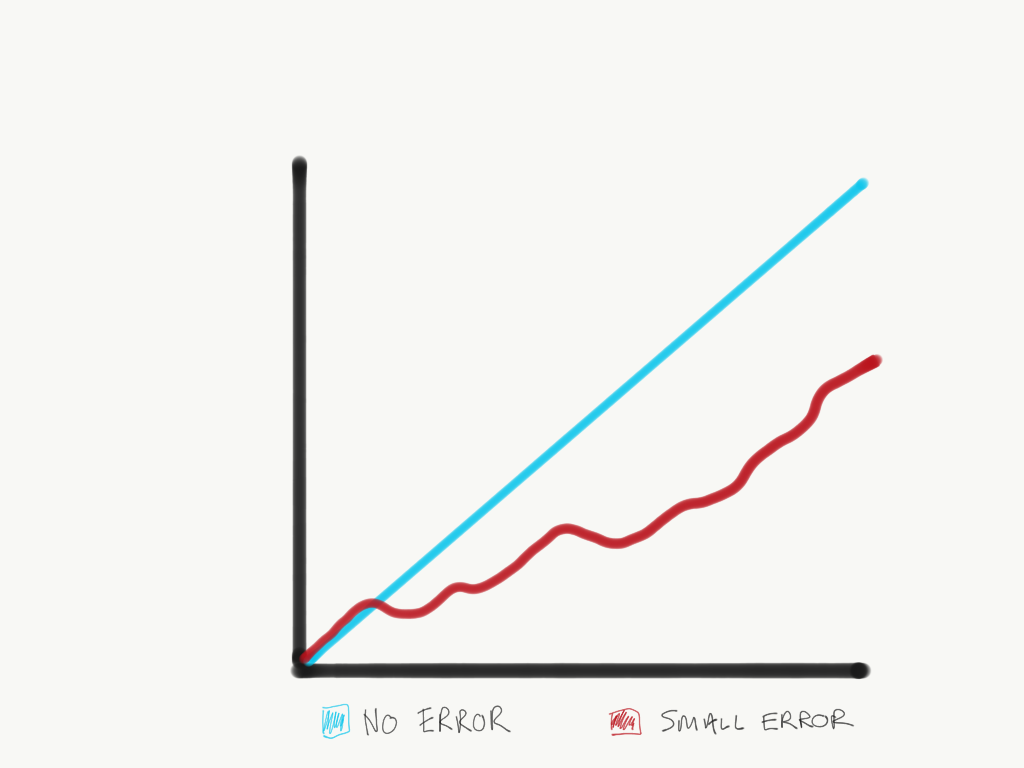
Have you ever thought about why forecasting snowfall is so difficult? Why can we seemingly ace temperature forecasts days in advance but can’t even nail down a snowfall forecast the night before a storm?
The Butterfly Effect
Snowfall is a puzzle in the forecasting world, with every piece tangled in complex atmospheric dynamics, chaotic patterns, and ever-shifting terrain influences. Predicting snow isn’t like projecting temperatures or wind speeds; it requires pinpointing two crucial variables: precipitation and temperature. Chaos theory helps us understand why this is so challenging. In a chaotic system like the atmosphere, tiny shifts in conditions can drastically alter the weather days later. A small fluctuation can turn a forecasted mild winter day into a snowstorm in just a few hours. This unpredictable behavior can make it feel like the atmosphere has a mind of its own.
To account for this chaotic nature, forecasters use ensemble models. Rather than one deterministic outcome, these models generate multiple scenarios to capture the range of possible weather patterns. Think of it as running the same forecast through different “versions” of the atmosphere to see how small changes affect the outcome. The result is a spread of forecasts that, when viewed together, give a fuller picture of what might happen. But even ensemble forecasting only goes so far, and many challenges remain, especially in mountainous terrain.
Model Resolution and the Terrain Problem

Global models like the GFS and ECMWF, built for large-scale patterns, run on grids that are too coarse for precision in areas with complex terrain. These grids cover tens of miles at a time, so they can’t capture the details of mountainous regions where elevation, slopes, and valleys change rapidly over short distances. When a global model sees the Rockies or Sierra Nevada, it’s essentially “blurring” their features, leading to snow forecasts that often miss the mark.
This is where downscaling comes in. With downscaling, we take these coarse forecasts and “sharpen” them to produce higher-resolution insights. Dynamic downscaling uses physics-based models to simulate the atmosphere on finer grids, while statistical downscaling leverages historical data to adjust outputs based on local conditions. These methods help capture the nuances of mountain weather, where snow forecasts can vary dramatically from one valley to the next. Yet, downscaling is only as good as the data and computational power behind it, and there’s always more ground to cover.
The Dual Challenge of Snowfall Prediction: Precipitation and Temperature
Snowfall forecasting is a balancing act. To predict snowfall accurately, models must get two things right: precipitation intensity and temperature. Even if a model nails the precipitation amount, a minor error in temperature prediction can shift a forecasted snowfall into rain—or worse, give an inaccurate snow depth. This is because snowfall depth depends on the snow-liquid ratio, or SLR for short, which describes the relationship between liquid water and the resulting snow.
SLR changes with temperature, humidity, wind, and more. Predicting it with precision is tricky because no universal formula exists. SLRs can range widely, especially in high-altitude regions or during cold, dry events. To predict snowfall, models must struggle between getting the precipitation amount and SLR exactly right—a difficult task even with today’s advanced techniques.
Uncertainty in Snow Liquid Ratio Equations

If you’re looking for a hard-and-fast formula to predict SLR, you won’t find one. Unlike wind or pressure, where we have equations that can describe behavior accurately, SLR lacks a universal law. Temperature, humidity, and atmospheric stability all interact, making SLR challenging to pin down; we simply don’t know exactly how atmospheric variables influence the SLR. These factors shift unpredictably, creating uncertainties in any snowfall forecast based on SLR estimates.
Formulas for SLR forecasting exist, such as the Cobb or Kuchera methods, but they are fairly unskilled.
Machine Learning and Enhanced Forecasting Techniques

Machine learning transforms snowfall prediction by addressing two major challenges: expanding ensemble forecasts and refining SLR predictions. With machine learning, models can generate more ensemble members quickly, simulating a wider range of possible outcomes without slowing down. These data-driven models can also perform downscaling tasks much faster, using patterns from past data to fine-tune high-resolution snowfall predictions based on terrain and atmospheric conditions; think of clicking a magic button that turns a low-resolution photo from your phone in 2008 to a stunning 4K image.
Data-driven SLR algorithms are another emerging area. These algorithms can infer more accurate SLRs than traditional formulas by analyzing years of snow and temperature data. Imagine a system that learns to adjust SLR based on real-time atmospheric inputs—temperature, humidity, and altitude—to give skiers and forecasters a better idea of how much snow will fall. It’s a step toward eliminating one of the major sources of error in snowfall forecasting and could bring a level of precision that today’s methods can’t match.
Conclusion: The Road Ahead for Snowfall Forecasting
The battle to forecast snowfall precisely is ongoing, but progress is being made. Chaos theory and coarse model grids will always pose challenges, especially over mountainous terrain. Yet, with advancements in machine learning and downscaling, we’re pushing closer to reliable predictions that account for terrain and atmospheric complexity. More accurate, data-driven SLR algorithms could one day remove some guesswork from snowfall forecasts. As the technology continues to evolve, skiers and forecasters may find that they can finally count on the snowfall forecast.