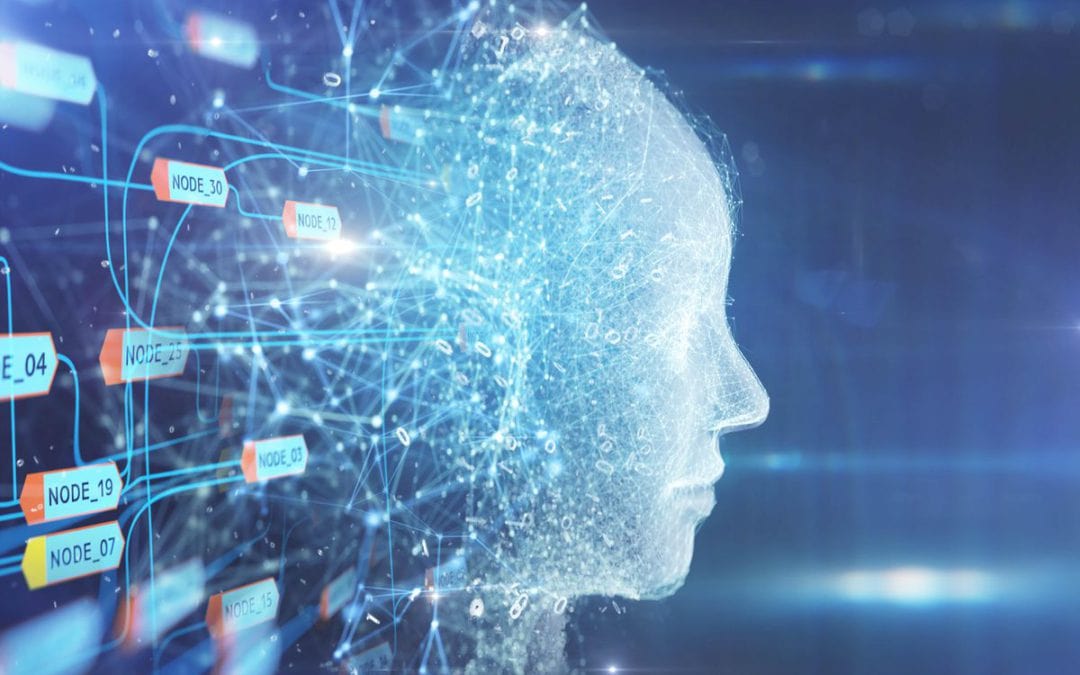
When is the next pow day going to be? That is a question that all of us would love to be able to answer. For ski areas, knowing when the snow will come is extremely important for almost every aspect of mountain operations, including when to groom runs and plow roads, when to do avalanche control work, and how many kegs to buy for the next pow day aprรจs. For skiers, knowing if there is a big storm around the corner could be the difference between another week at work and an impromptu ski trip. Artificial Intelligence (AI) is being used to solve larger and larger problems every single week, but can it be used to tell us when the freshies are on their way?
Googleโs DeepMind project recently released GraphCast, a machine learning-based weather forecasting software focusing on medium-term forecasts (3-10 days). GraphCast provides forecasts anywhere on the planet in six-hour time increments, similar to state-of-the-art Numerical Weather Prediction (NWP) models like the Global Ensemble Forecast System (GEFS). One key advantage of GraphCast is that new forecasts can be generated 1,000 โ 10,000 times faster than NWP models, which take hours of supercomputer time to propagate the numerical model forward in time to generate new forecasts.
In a paper published earlier this month in the journal Science, the DeepMind team reported that GraphCast delivers a more accurate forecast than the HRES in >90% of cases. This increase is because of the extensive training and optimization of a Graph Neural Network, a machine learning technique, which used almost four decades of global weather data to set up the model. This training process took 32 state-of-the-art supercomputers from Google Cloud close to four weeks to crunch the numbers! The result is more accurate weather forecasts delivered in a fraction of the time.
Despite the excitement around GraphCastโs release, there are questions as to whether or not GraphCast will be able to improve mountain weather forecasts, especially snowfall. Mountainous regions have a few different features that make weather forecasting notoriously difficult. The rapidly changing elevations of mountain ranges can get lost in the course grids most weather models, including GraphCast, use to represent the Earth. Moreover, specific topographies such as ridges and valleys can have drastic local effects on temperature, wind, precipitation, and other factors. One of the biggest challenges for using large machine learning models like GraphCast for snowfall in the mountains is that snowstorms in the mountains are relatively rare events in both time and space, meaning that the neural network has fewer examples of these weather events to learn from. Put simply, GraphCast may not be any better at predicting snowfall, because it was not optimized to predict snowfall.
Many current weather models overestimate storm totals because of the difficulty in predicting the snow-liquid ratio. This ratio is responsible for determining whether snowfall will be blower pow or thick mashed potatoes. Current weather models can predict the amount of moisture that a storm is likely to produce, but they often assume a fixed snow-liquid ratio when determining how much snow will accumulate. Recent research from the University of Arizona trained several different types of machine learning models to better predict the snow-liquid ratio and forecast snowfall in the mountainous regions of Arizona and New Mexico. They highlight some of the challenges of mountain forecasting in their paper, stating โErrors tend to be slightly higher near the peaks of mountains, [โฆ] lower elevations would have few events with relatively similar setups, ideal for a machine learning model, while the higher frequency of mountaintop events and the wider range of viable conditions for snowfall make prediction more challengingโ. AI will probably have more of an impact on predicting mountain weather in these smaller, specific projects, as opposed to large scale models such as GraphCast.