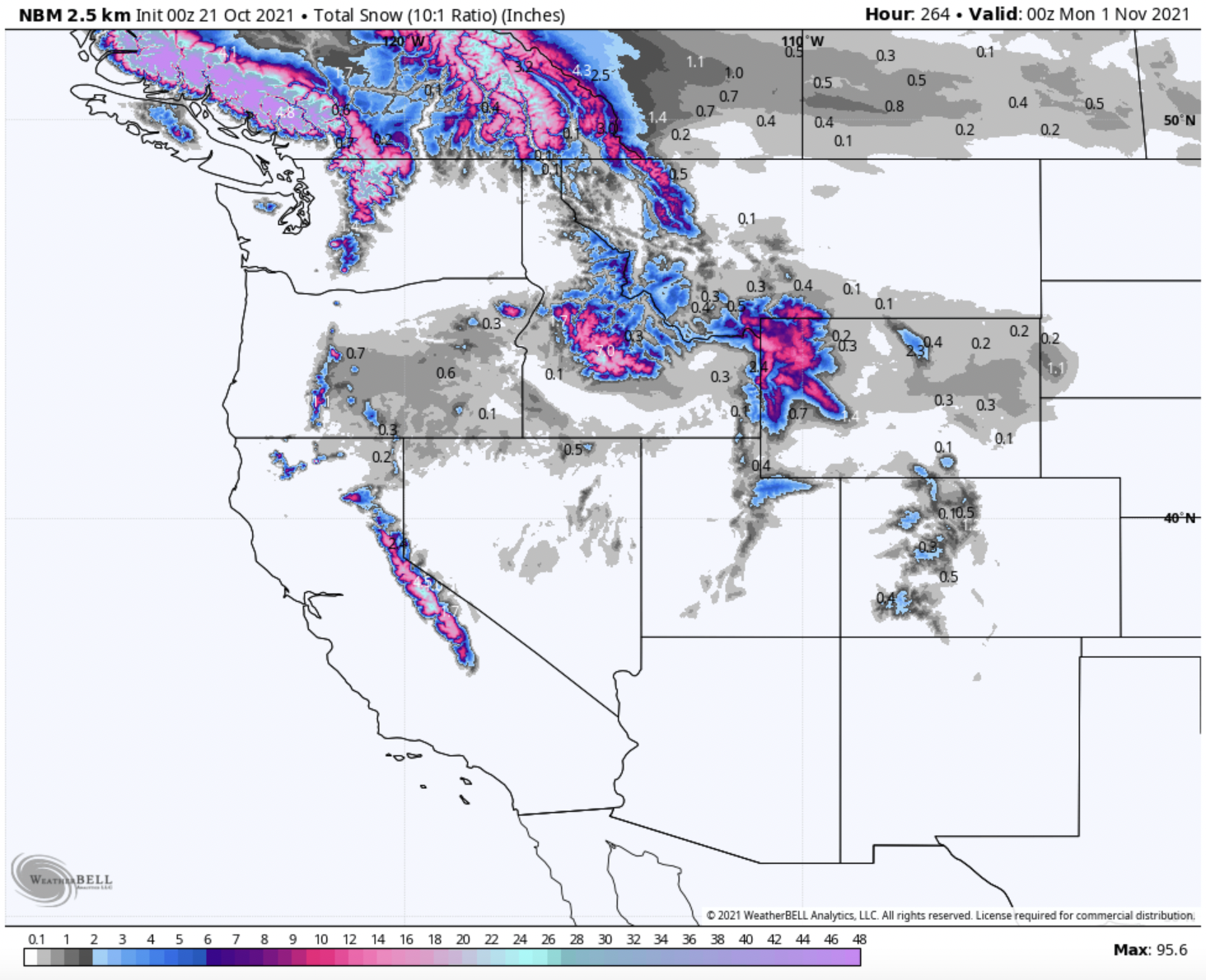
One of the most frustrating aspects of skiing can be the weather forecast. Your favorite weather app forecasts 12″ overnight, and you wake up the next morning, excited for a great day of powder skiing. However, much to your dismay, only 3″ has fallen! So, what went wrong?
Weather models take inputs from satellites, weather stations, and other forms of meteorological monitoring. This data is then assimilated with each new model run as the “starting conditions.” From the current conditions, the model gives its best guess of what will happen with temperature, precipitation, etc.
Deterministic forecasts attempt to nail down the exact conditions at any given point in time during the forecast. While this forecasting style is often excellent in the short term (within a few days), it typically breaks down after a few days due to the butterfly effect.
This is where ensemble forecasting comes in. An ensemble forecast is a group of “members” of the same model, but each member has slight tweaks to initial conditions. Ensemble forecasting helps us understand the most probable outcomes from weather events. Ensemble forecasts are much better at longer-range forecasts than deterministic forecasts are.
Each ensemble member is slightly different from all the others. Each member has slightly different initialization parameters, and while they generally agree with each other in the near term, these small changes in the initial conditions have big consequences even several days out. By tweaking the start conditions, ensemble forecasting strives to account for chaos theory by forecasting a wider range of possible outcomes.
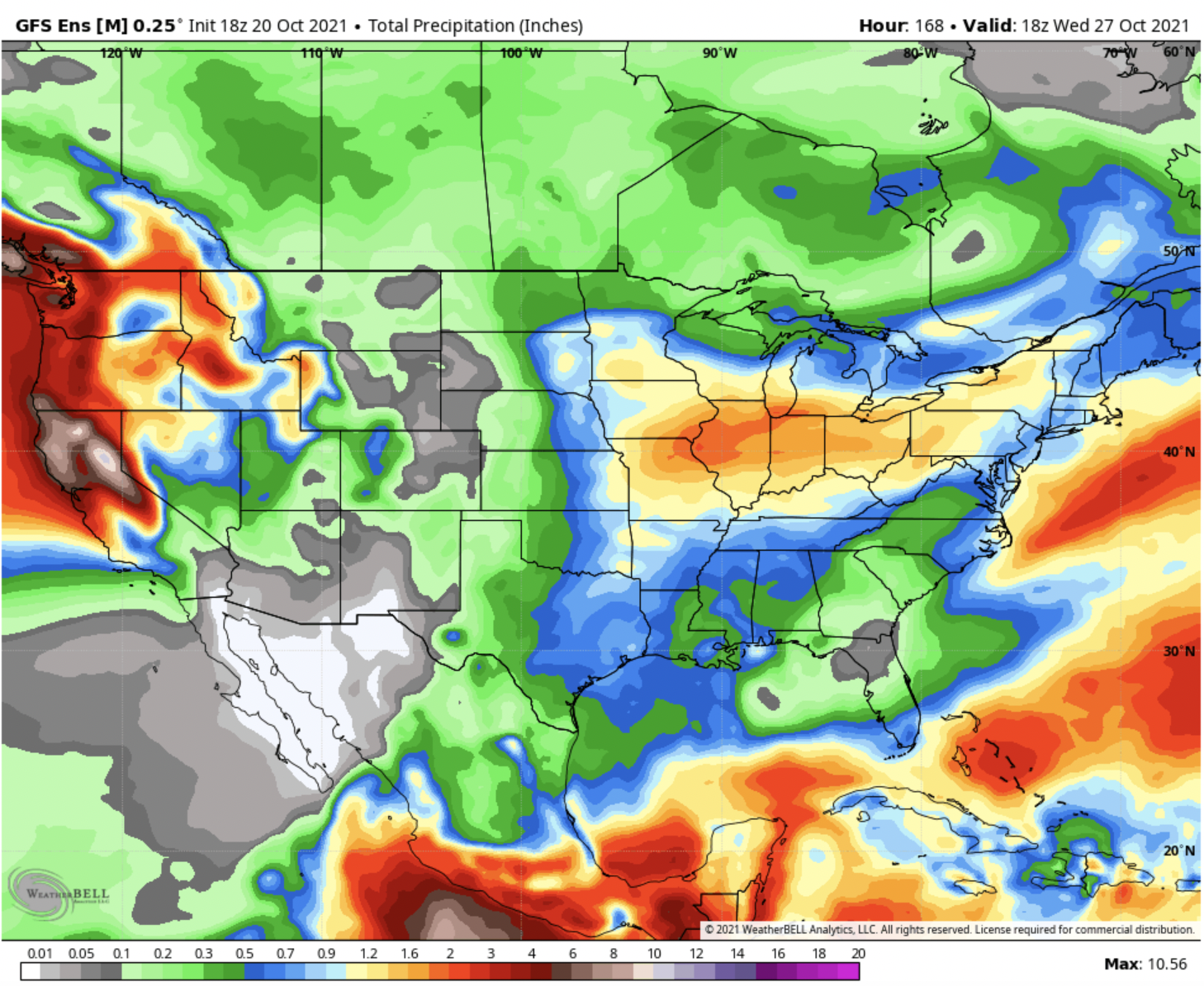
Ensemble forecasts can have three different outputs. The first is quite similar to deterministic forecasting. The ensemble mean is the average of every ensemble member combined. This is good for pinpointing where the highest precipitation accumulations will be in the medium-range forecast period. The more the members agree, the “sharper” the forecast becomes; the less agreement, the more messy and unorganized.
To see what “sharper” means here, let’s say we have an ensemble of 50 members. In a hypothetical scenario, 25 members think a storm will hammer the PNW, and 25 members think a storm will hammer further south in California. The ensemble mean would indicate equal precipitation totals in both regions, which would dull out the overall precipitation forecasts for both regions (if the average of the 25 PNW members were 3″ of precipitation, the overall ensemble mean would be 1.5″, thus a “duller” forecast).
Now, in the same hypothetical 50 member ensemble, we have all 50 members agreeing on the PNW to be favored. Let us say that the average of the 50 PNW members is 3″. Now, since we don’t have any members bringing the ensemble average down with 0″ QPF forecasts, the ensemble average is 3″, even though the initial PNW members were still forecasting 3″ of precipitation! In other words, the more member agreement there is, the tighter and more organized the model output will be.
The second output is ensemble-derived probabilistic forecasting. Probabilistic forecasting aims to predict the chance that an event will occur rather than the exact outcome. For example, if all 50 ensemble members agree on 2″ of snow or more, the probability is 100%. If 37 members agree on 3″ or more, the odds become 74%, and so on.
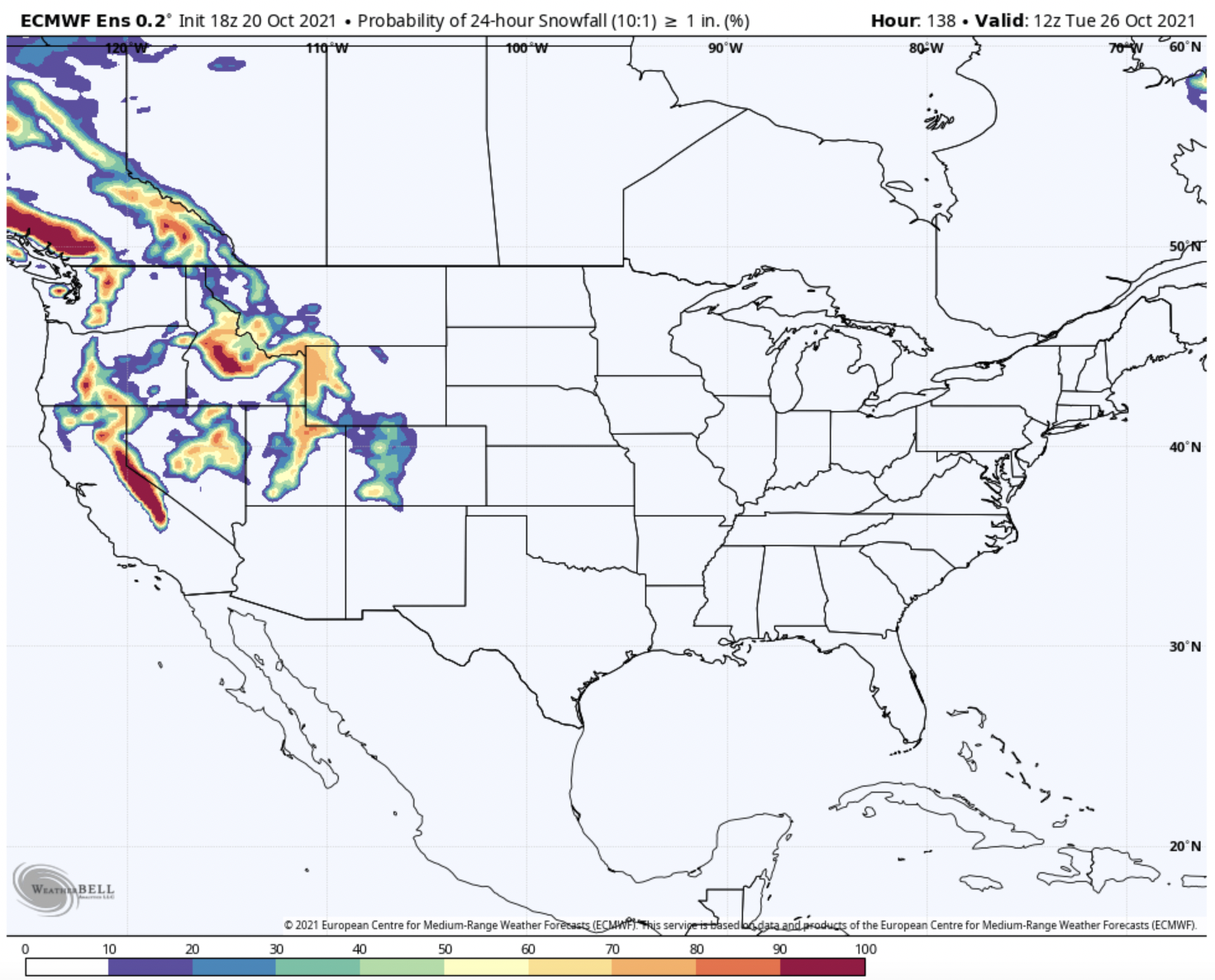
The final output is viewing individual member outcomes. This essentially treats each ensemble member as a deterministic model. Then you can view each one to see where they agree and where they don’t.
While it might not be particularly satisfying because it often does not pin down specific values, ensemble forecasting is a fantastic tool that meteorologists have to predict the most likely outcomes!
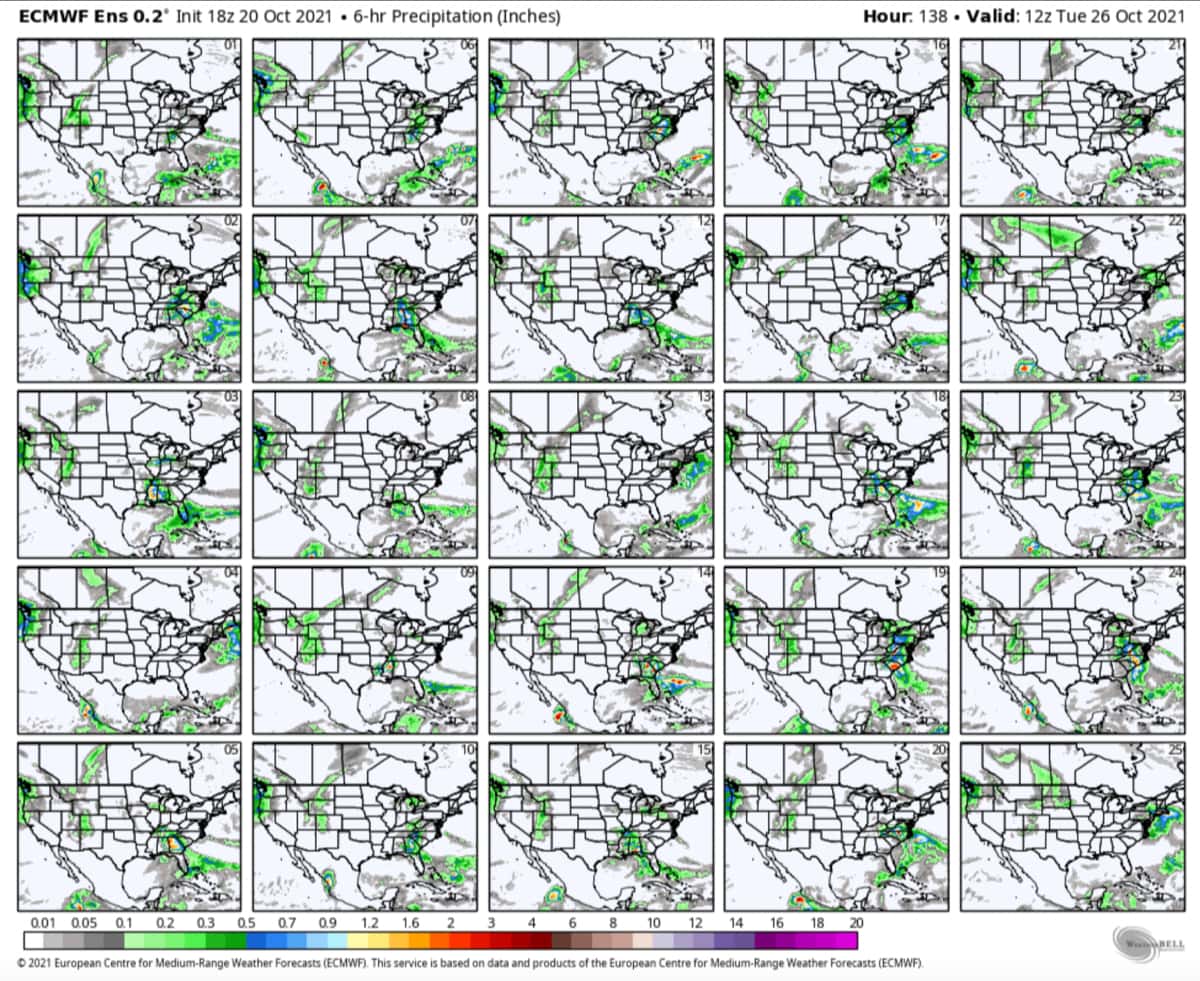
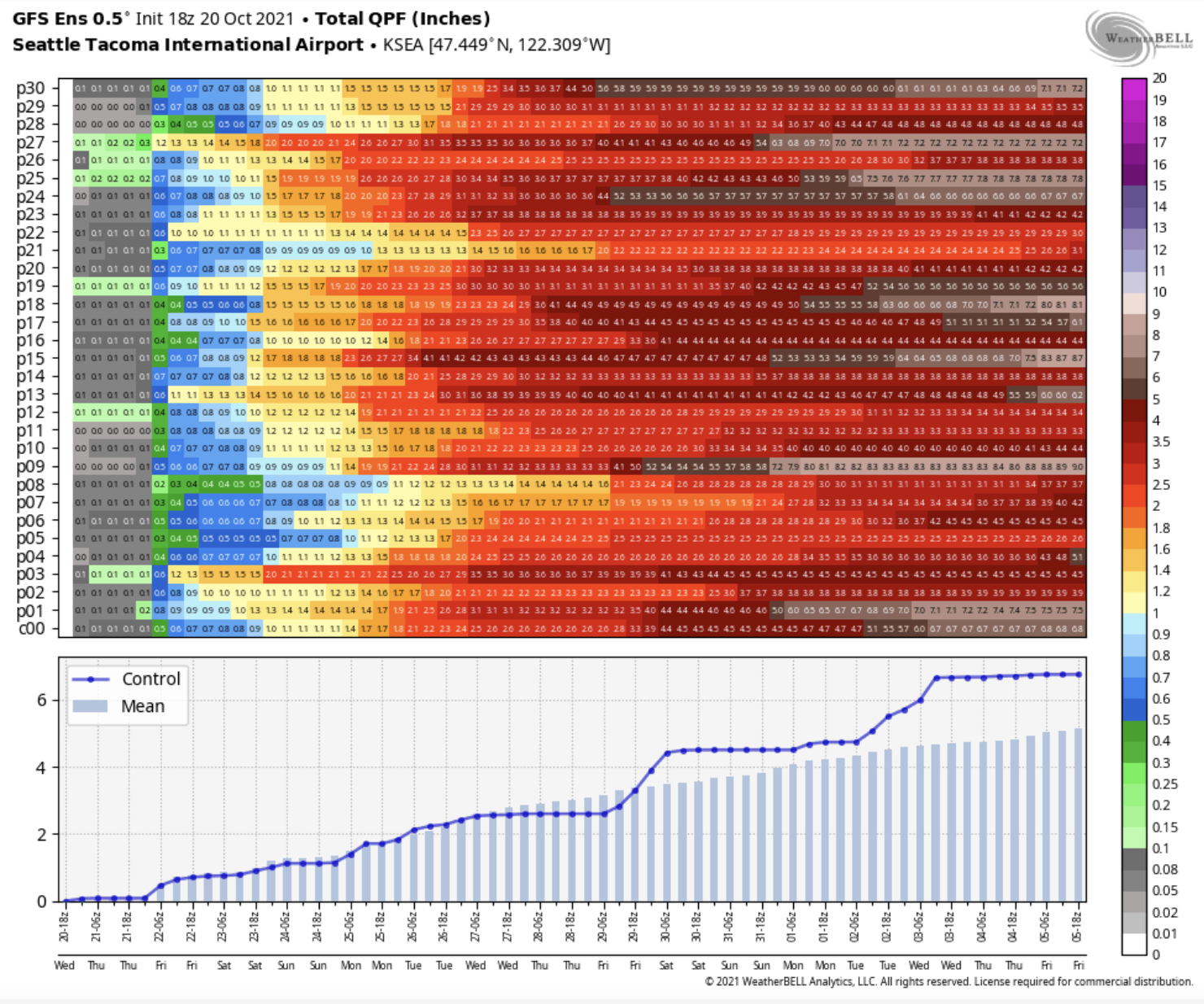